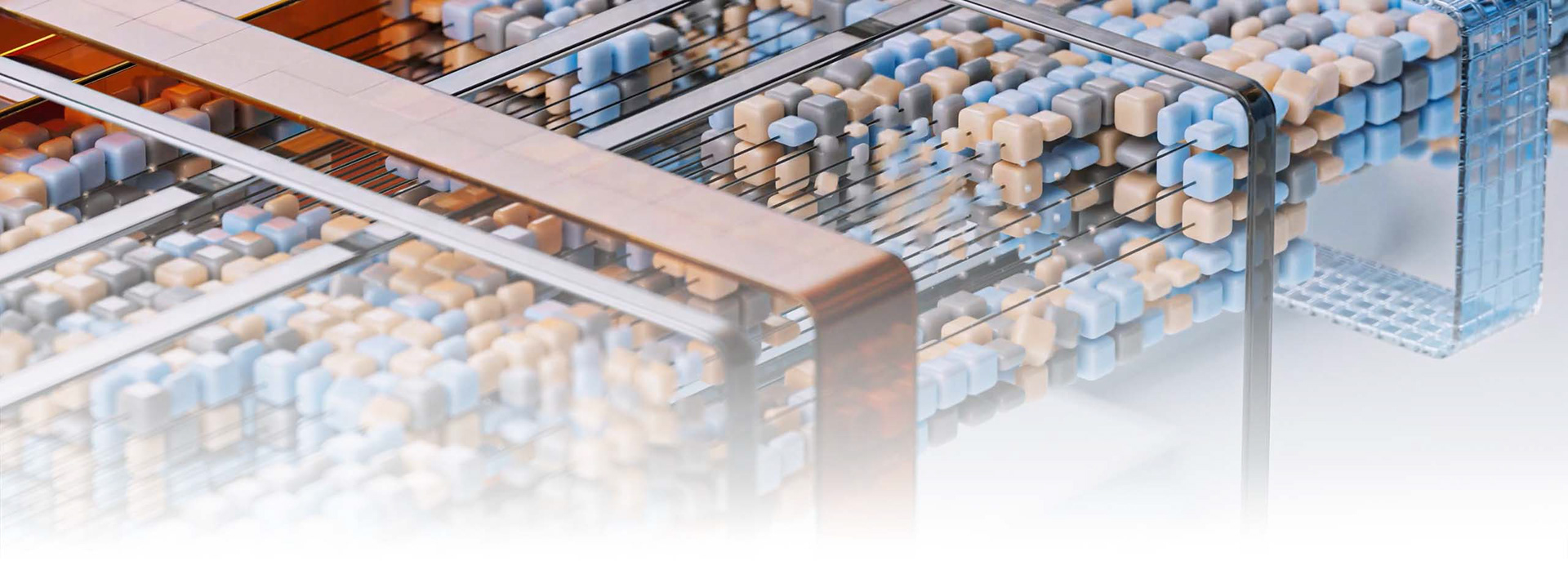
Visualizing AI is an initiative by Google DeepMind that aims to open up conversations around AI. Commissioning a diverse range of artists to create open source imagery, the project seeks to make AI more accessible to the general public. Visualizing AI explores the roles and responsibilities of the technology. It weighs up concerns and the societal benefits in a highly original collection of works by world-class creators. From the abstract to the literal, each image is an authentic representation of the artist’s take on AI. Available as part of an open-source library, the collection aims to shift dated perceptions of AI in order for everyone and anyone to have a say on the future of the technology.
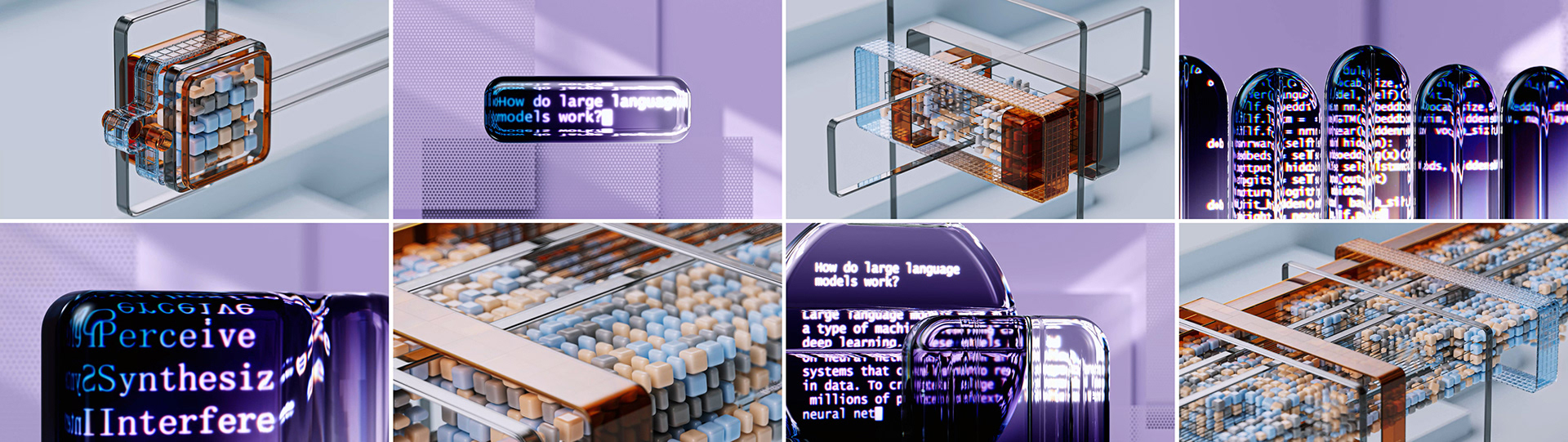
AI as depicted in popular culture is often associated with dystopian narratives that exclude the realistic risks and potential rewards of the technology. Visualizing AI began as an effort to redress this by commissioning artists to create imagery representing a more multi-dimensional picture of how AI can impact society. By doing so, the project aims to enable more discussions surrounding the subject, encouraging wider conversations and in turn, giving more people a voice in how the tech is developed.
Google DeepMind did not want to be prescriptive about the visual outputs of the project and wanted to ensure that there is a common understanding of the values that they wanted to communicate through them. Rather than providing moodboards & references, I was matched up with a scientist on each subject intended to elaborate on the philosophical values that were important to carry through in the animations that were created.
deepmind.com
deepmind.com

Large Data Models
Our initial collected data is being visualized through small cubes. While being used as a training set for the data model, the data exhibits a soft, glitchy movement. A grid is used to constrain the data during the evaluation process, as underlying relationships within the data are recognized.
As additional data is collected and analyzed, the model becomes increasingly complex, expanding over time within the animation. Through an iterative process, the previously constraining grid now moves more quickly through the collected data and is refined to recognize underlying relationships with greater accuracy and performance.
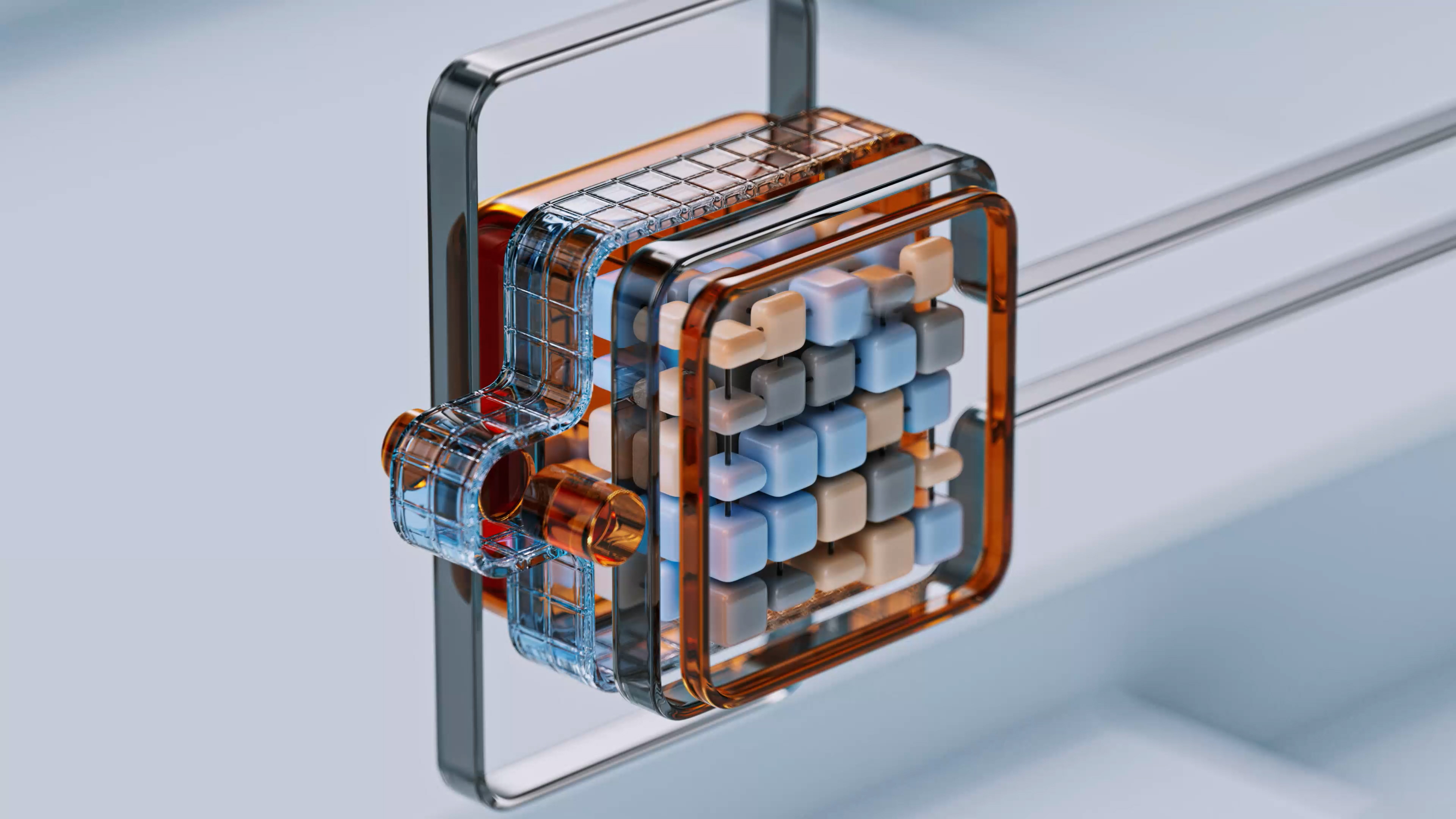
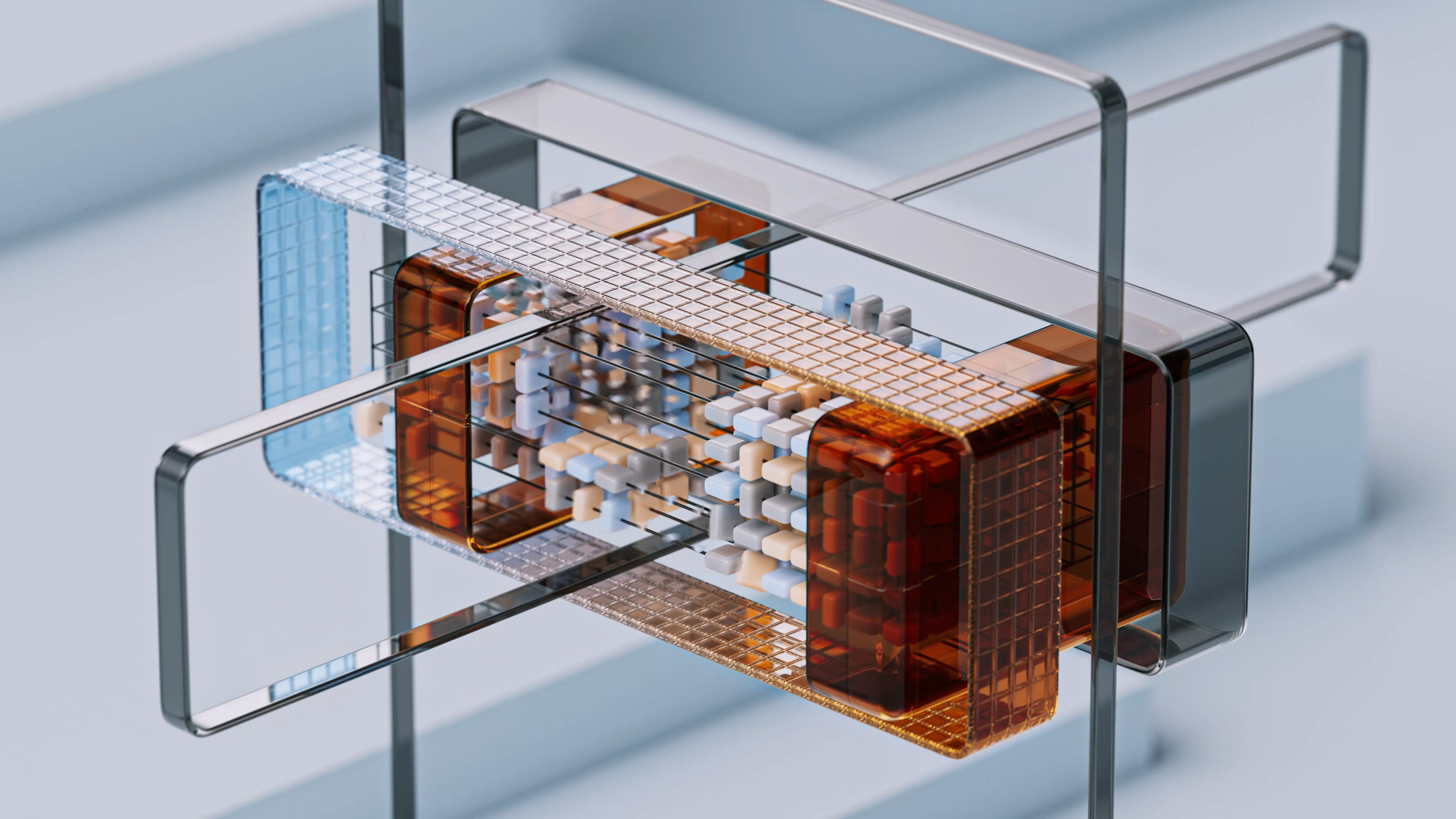
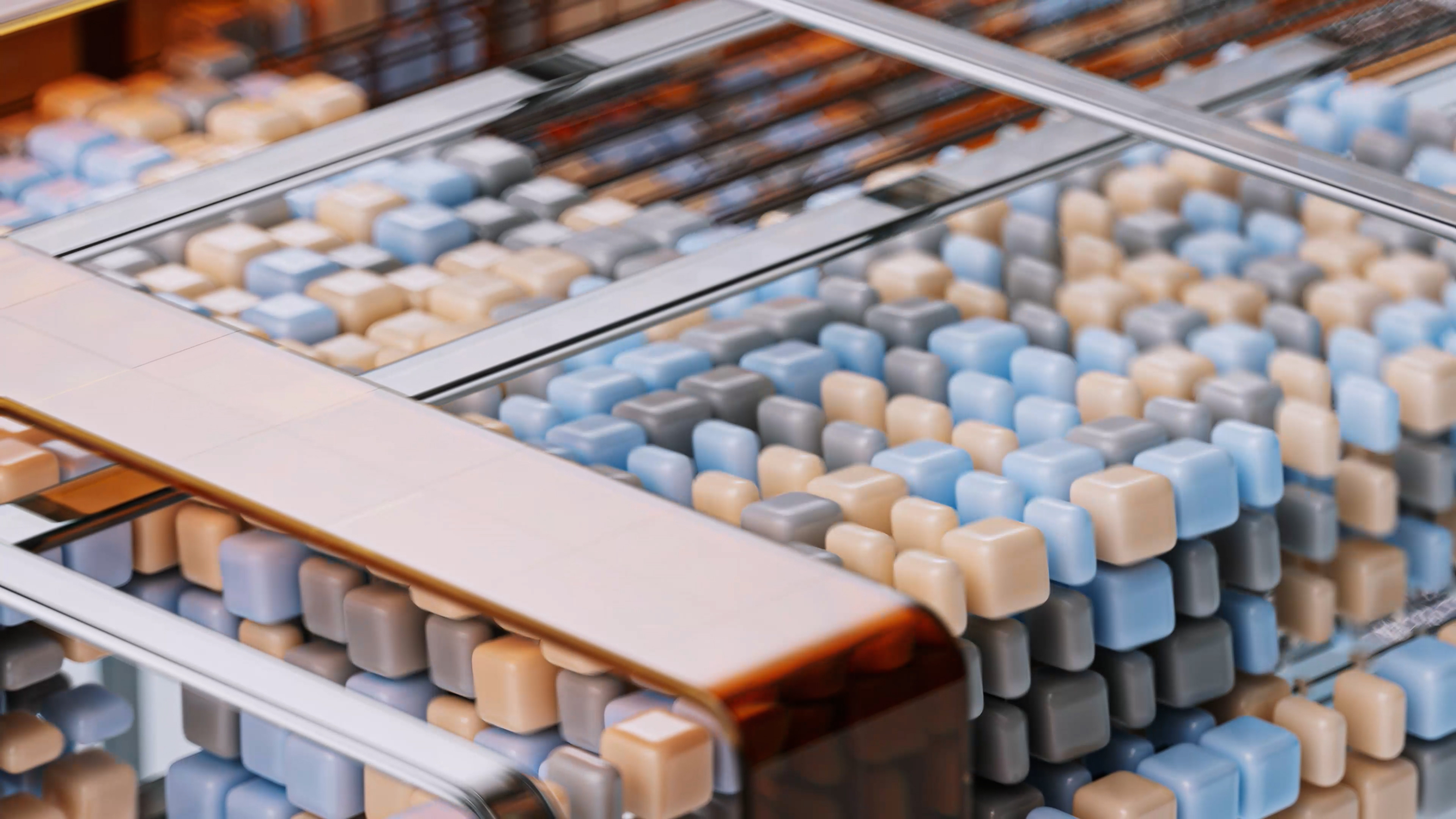
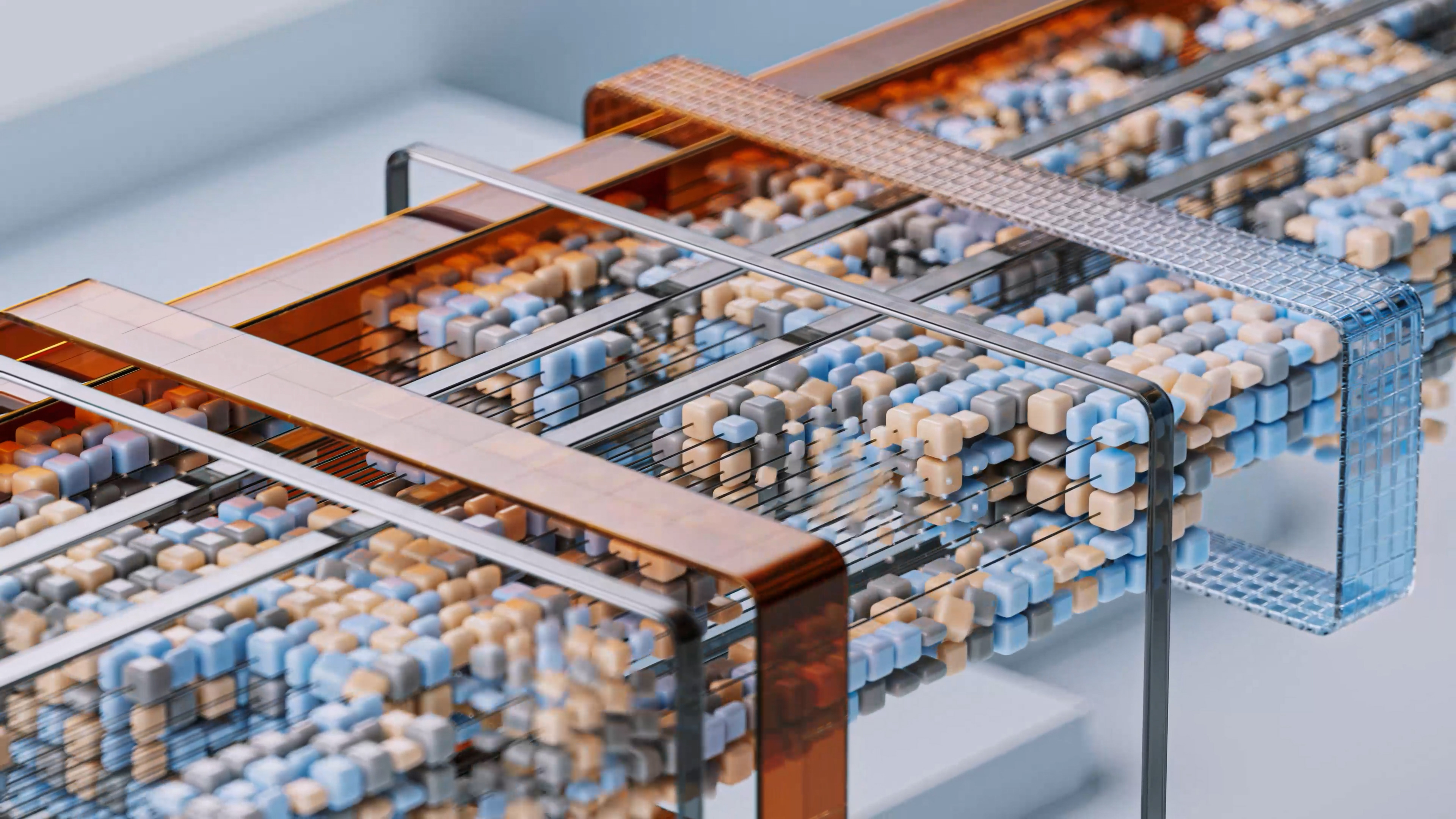
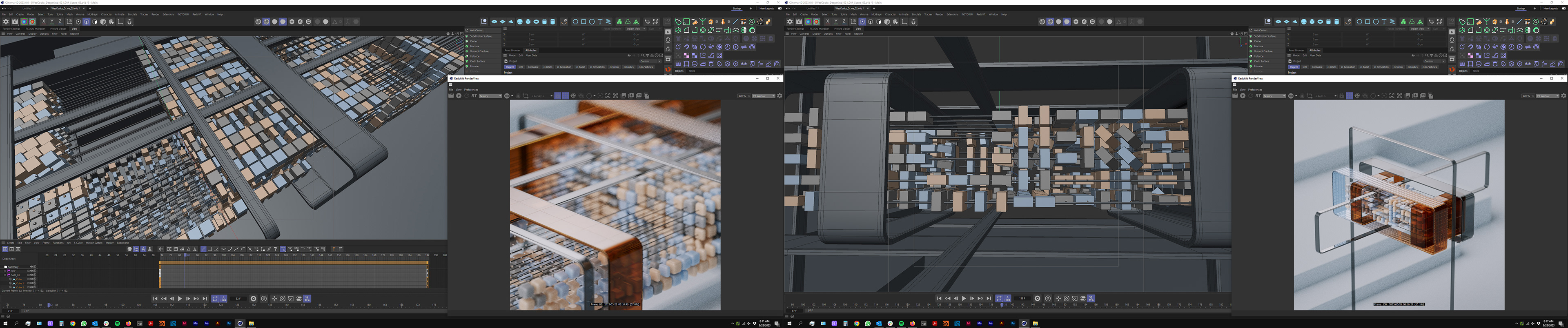

Large Language Models
This animation draws inspiration from large data models like Bard, ChatGPT, and others. It starts with a prompt that inquires about the workings of large data models. To dissociate from autonomous machine control and limit the subject matter to human usage, the shapes utilized evoke products that are not associated with any actual products. As the neural network traverses over scattered shards, it identifies statistical patterns and correlations among words and phrases.
This animation draws inspiration from large data models like Bard, ChatGPT, and others. It starts with a prompt that inquires about the workings of large data models. To dissociate from autonomous machine control and limit the subject matter to human usage, the shapes utilized evoke products that are not associated with any actual products. As the neural network traverses over scattered shards, it identifies statistical patterns and correlations among words and phrases.
The process involves perceiving, synthesizing, and interfering with the data. Once the answer to the initial question takes shape, the animation displays a sequence of synonyms for three keywords: perceive, synthesize, and interfere. This is achieved by computing a probability distribution across all possible next words or sequences of words. The network then randomly selects a word or sequence by sampling from that distribution to generate the next word or sequence.
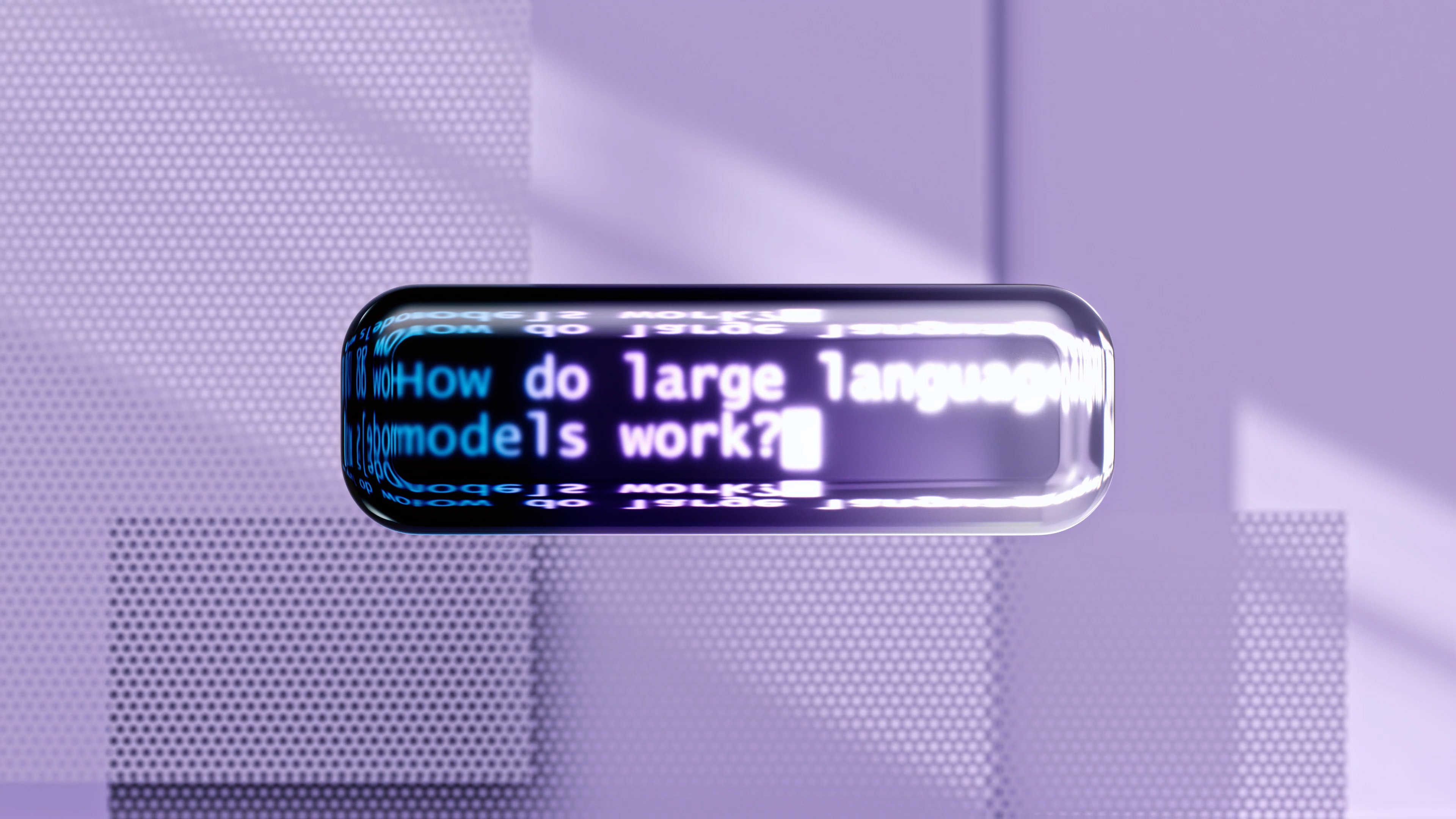
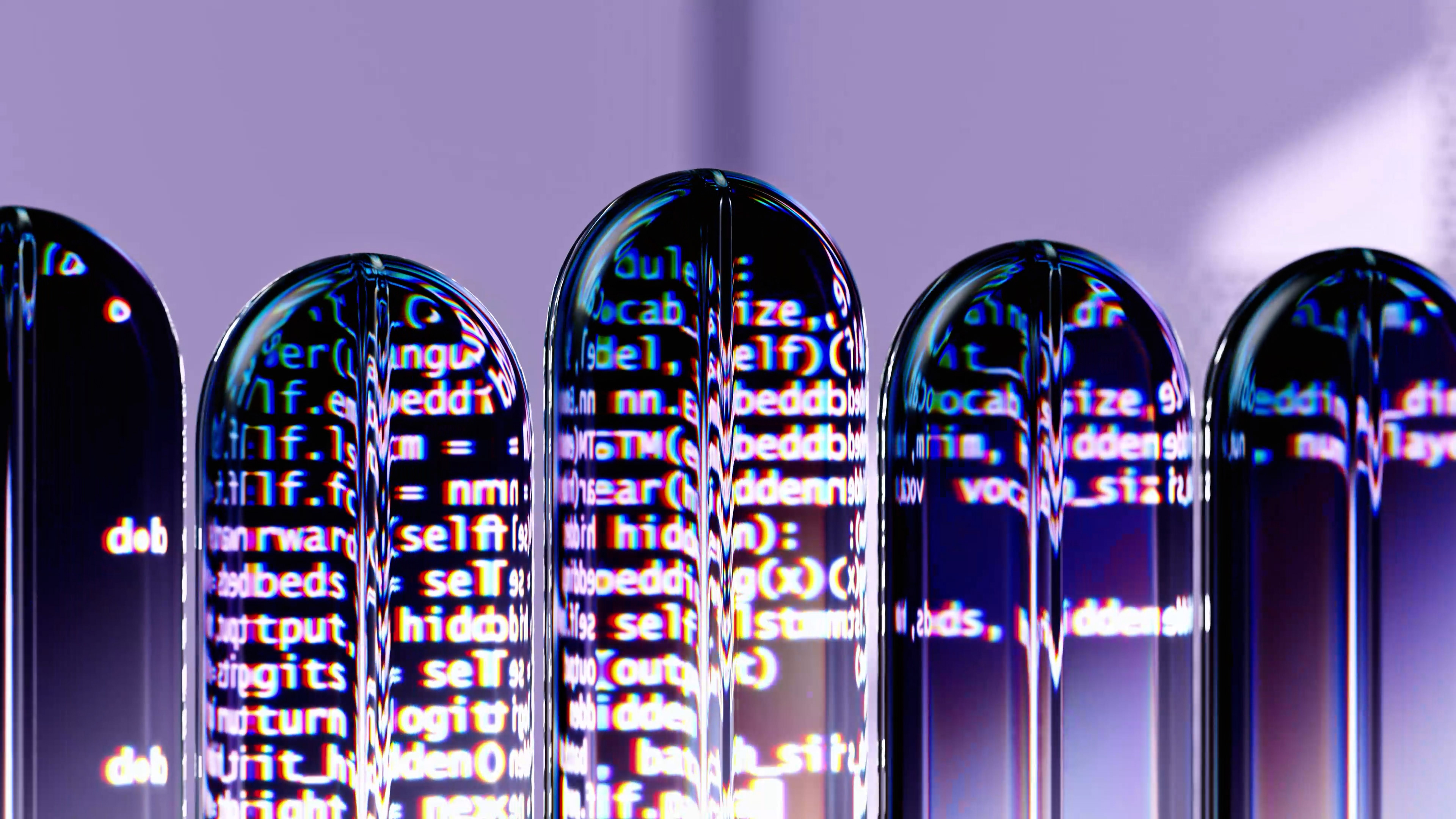
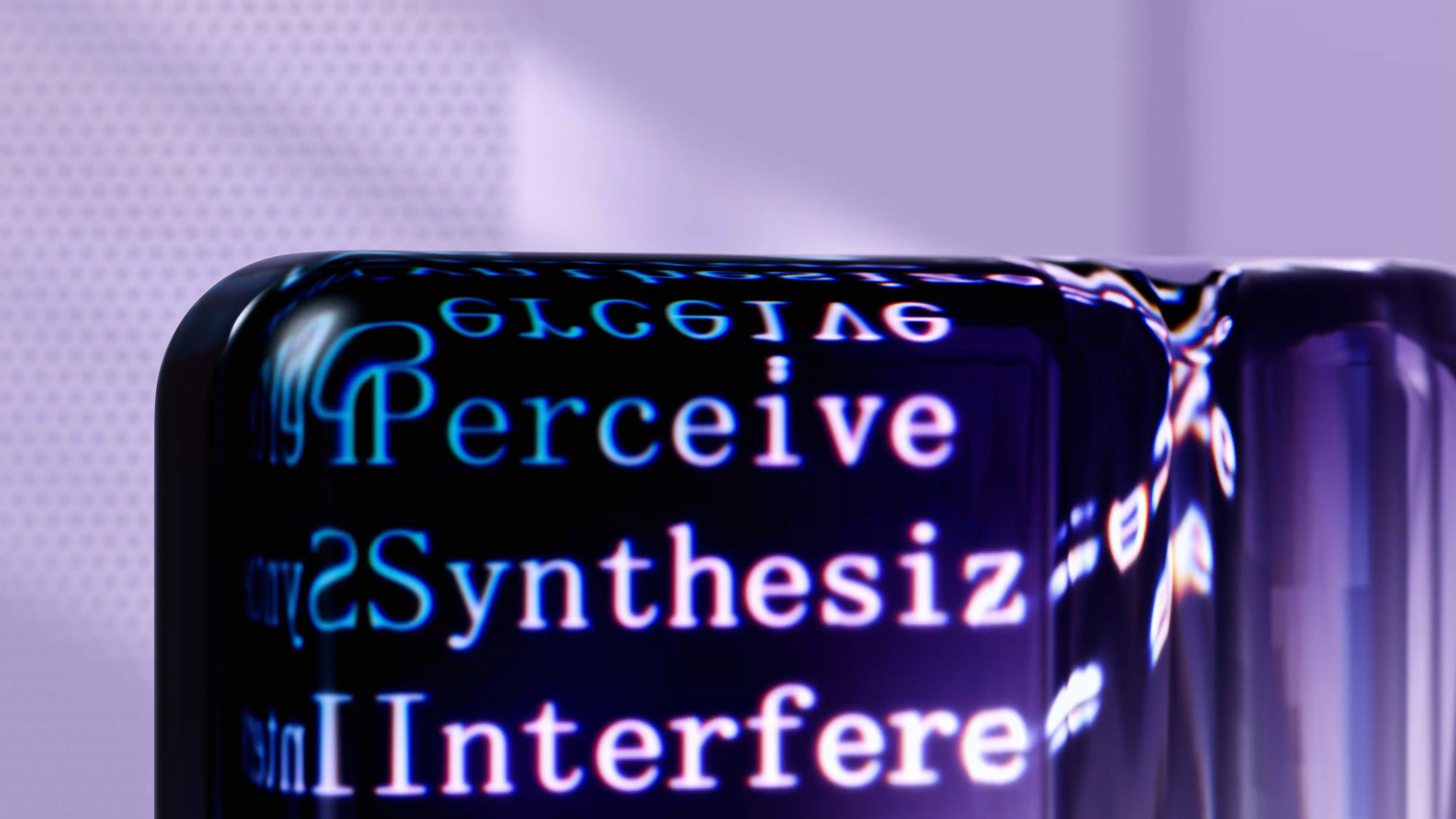
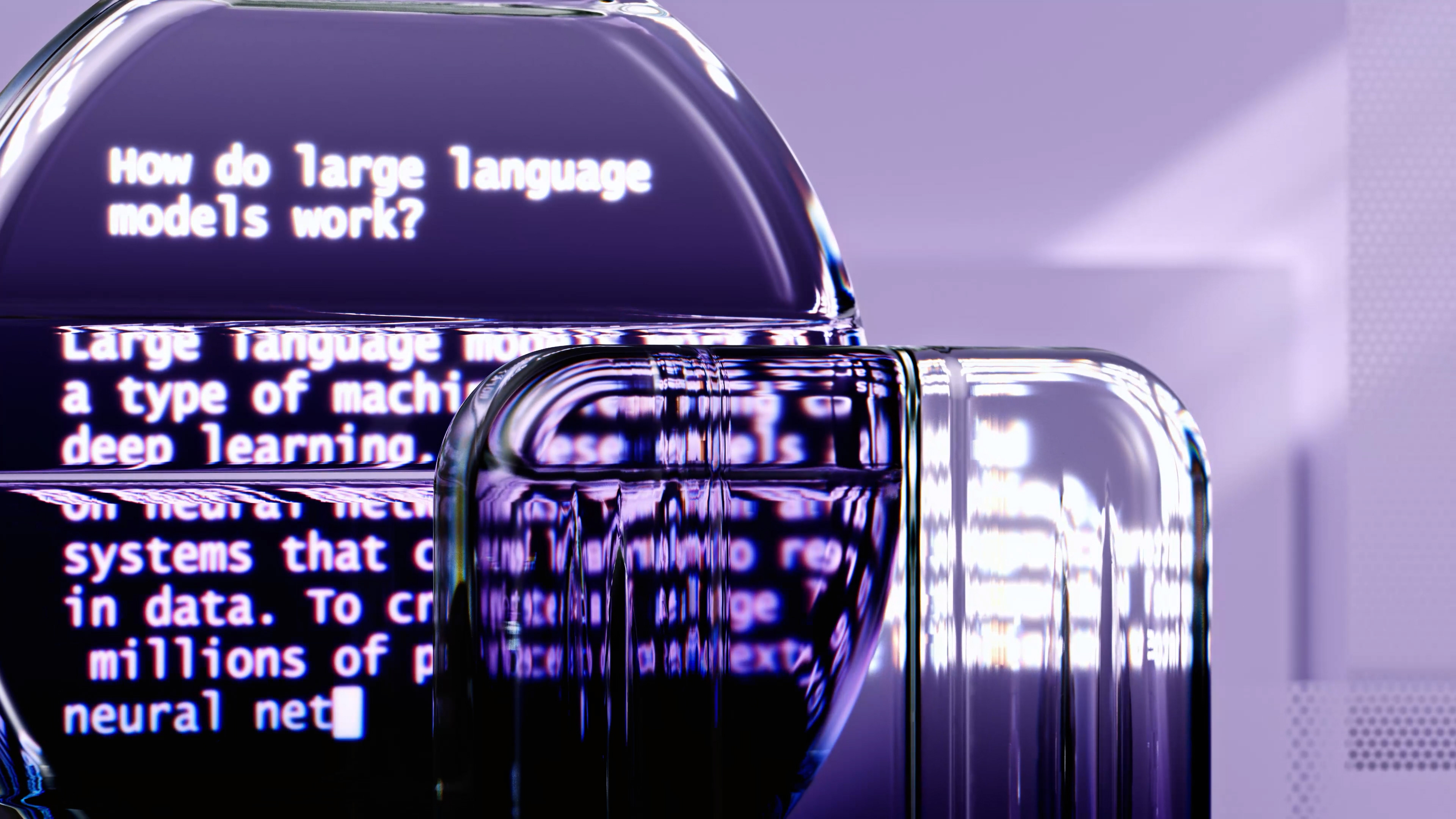
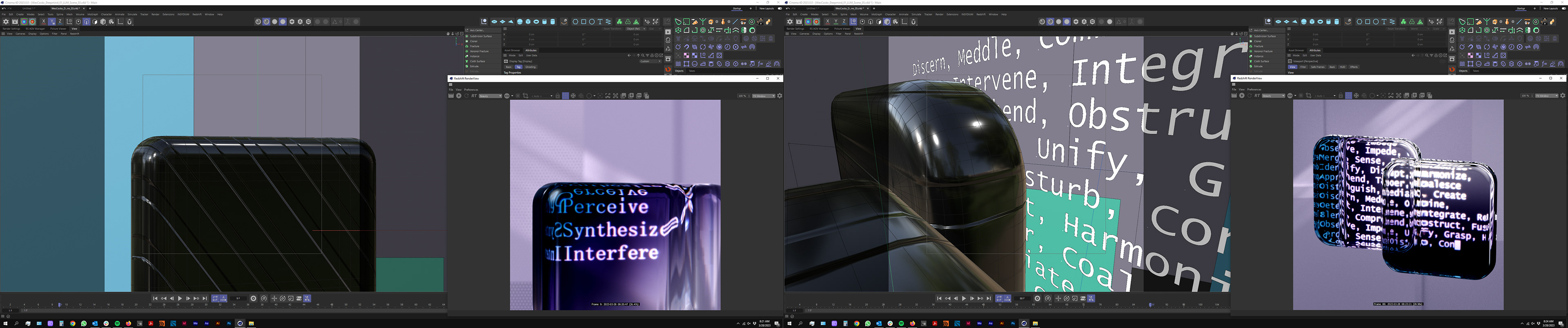

RnD & Process
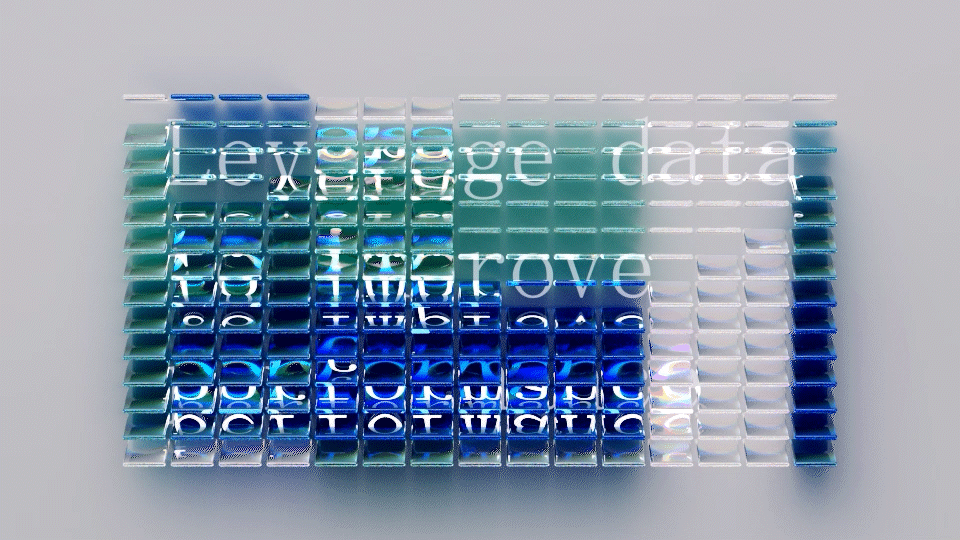
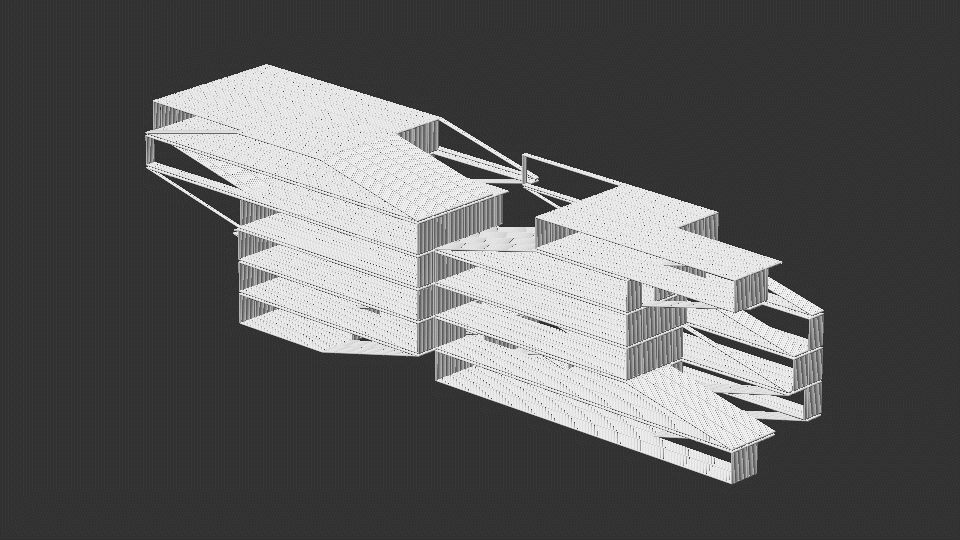
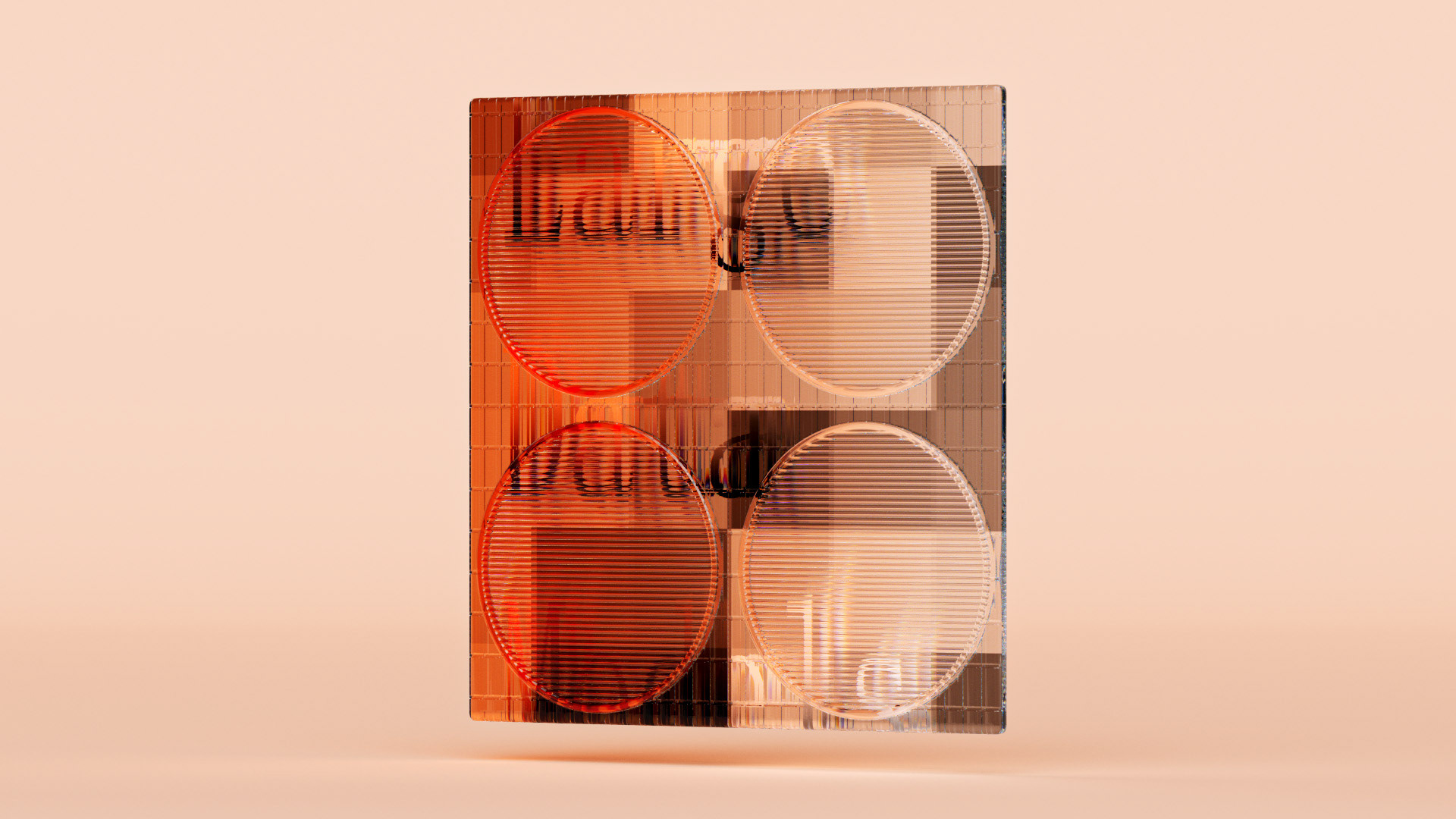
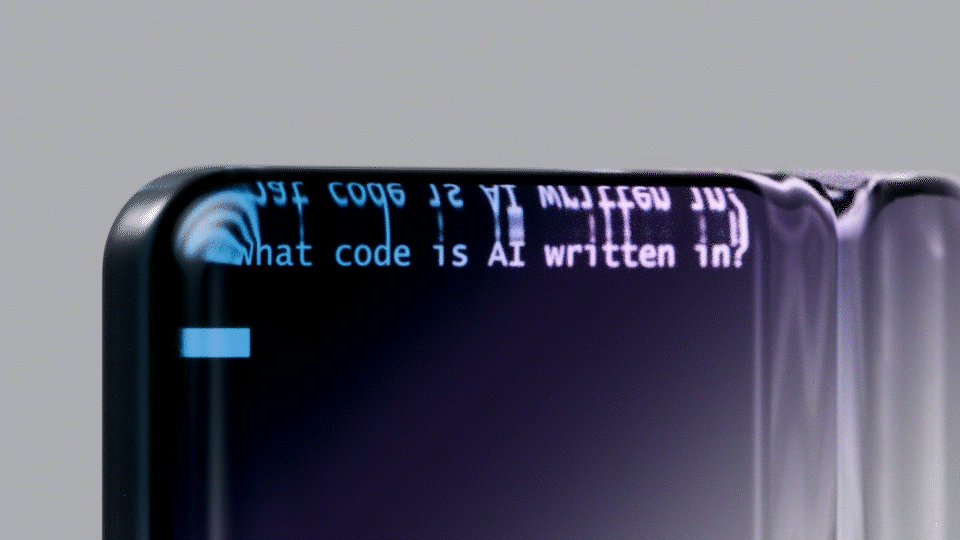
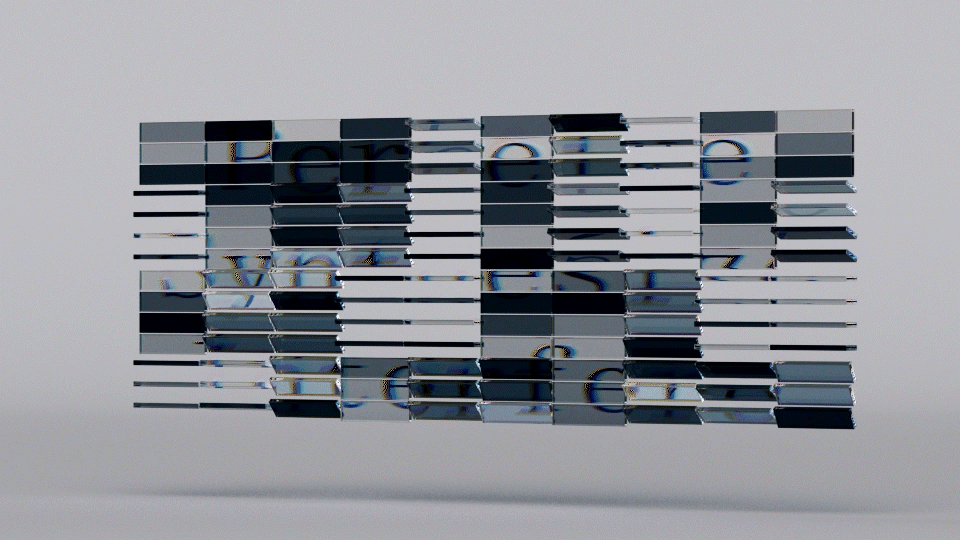
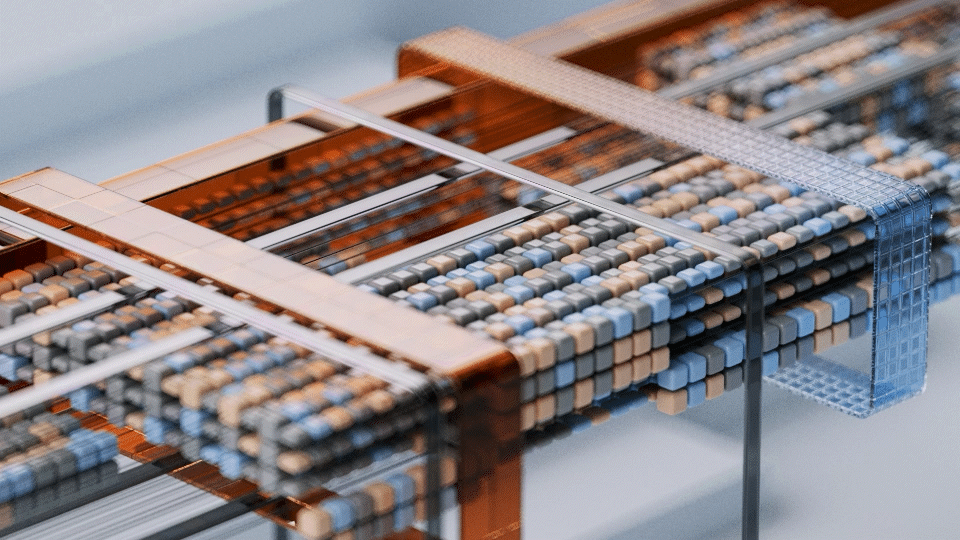
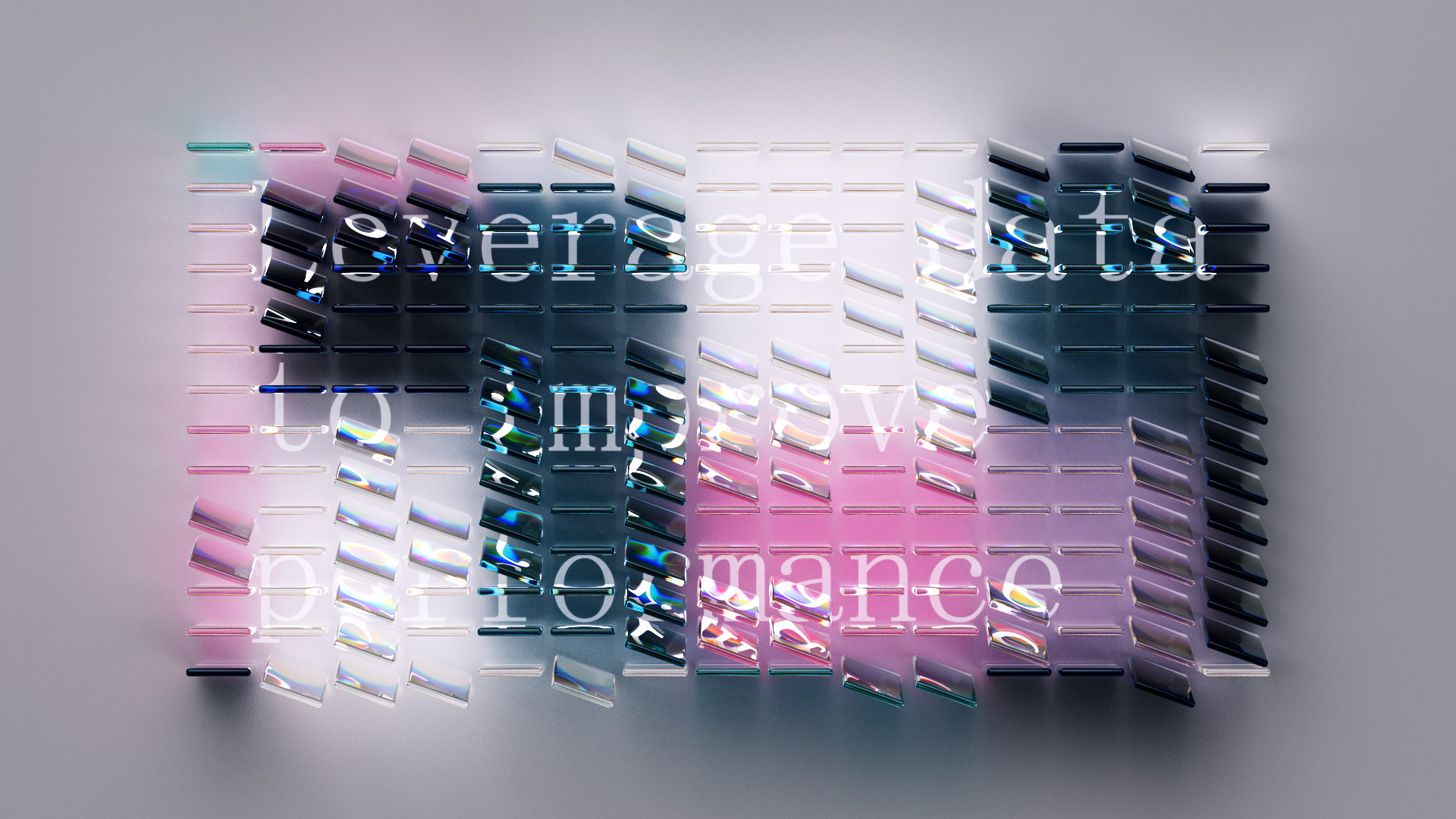
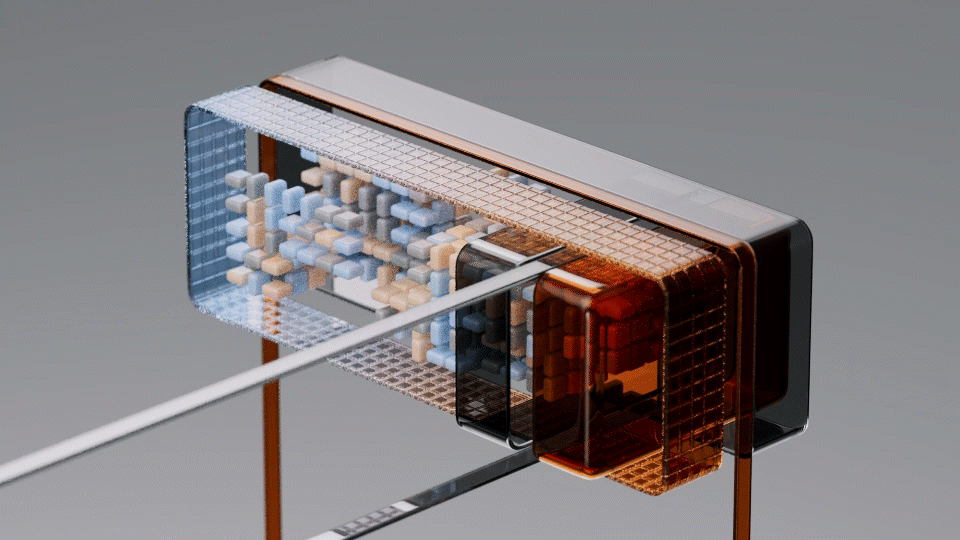
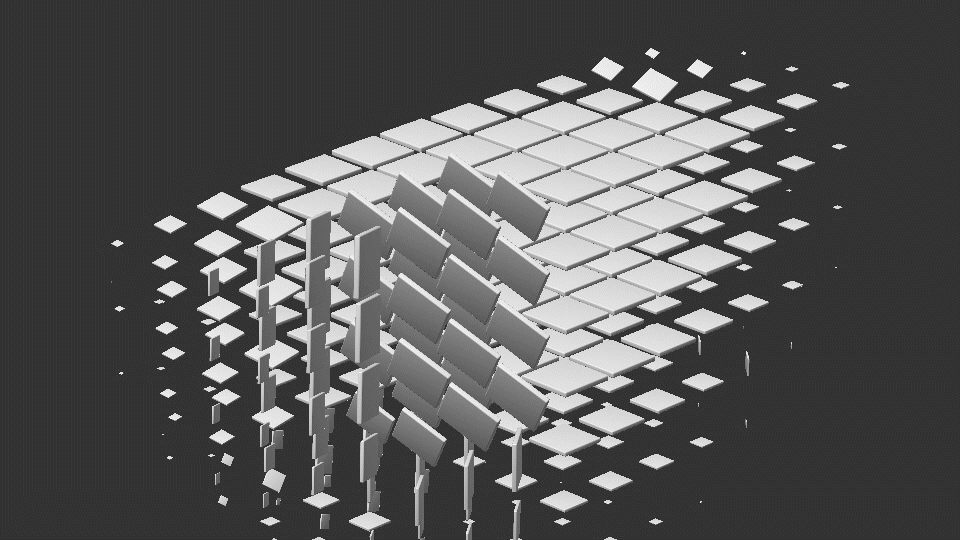
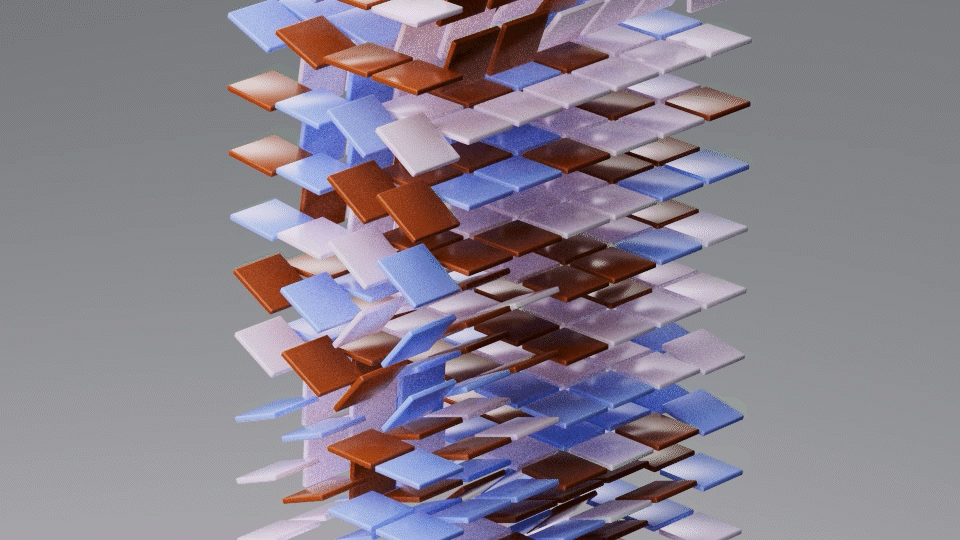
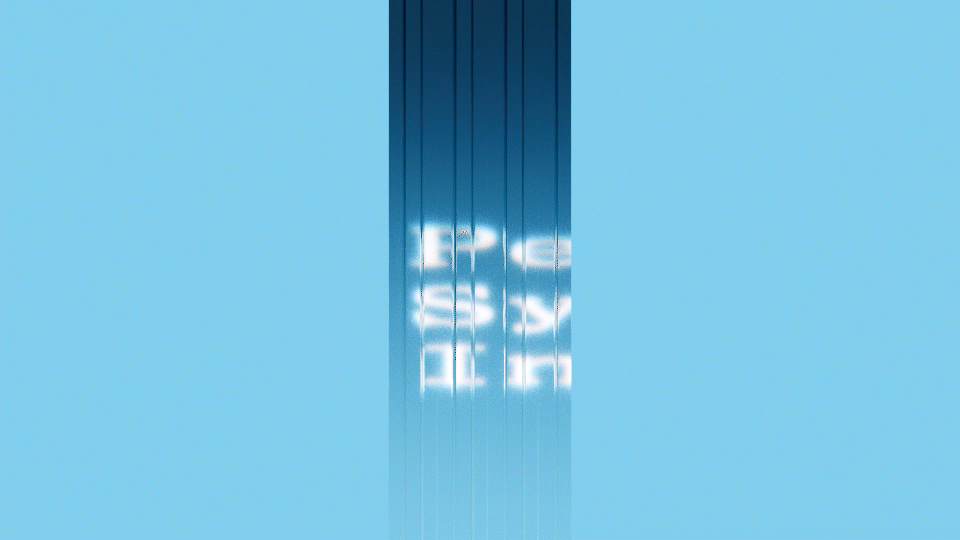
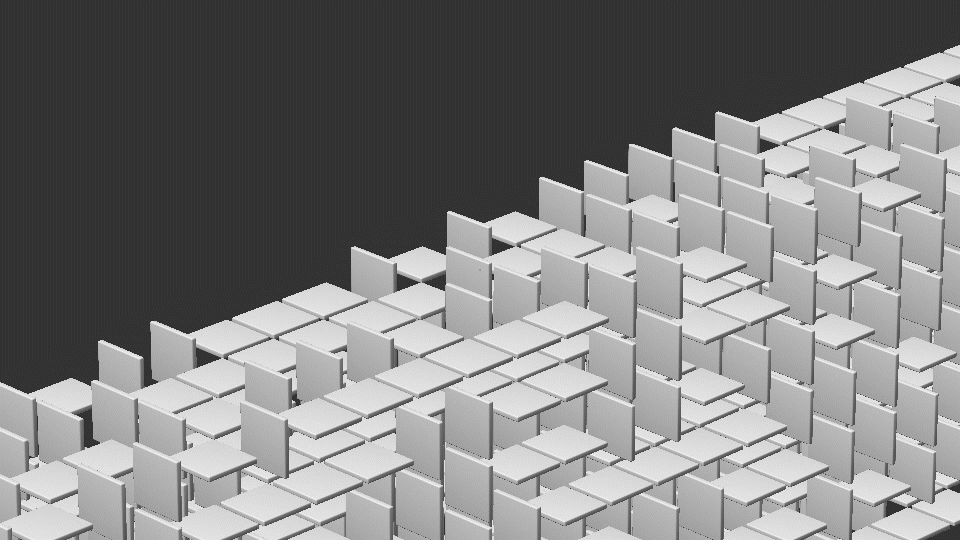
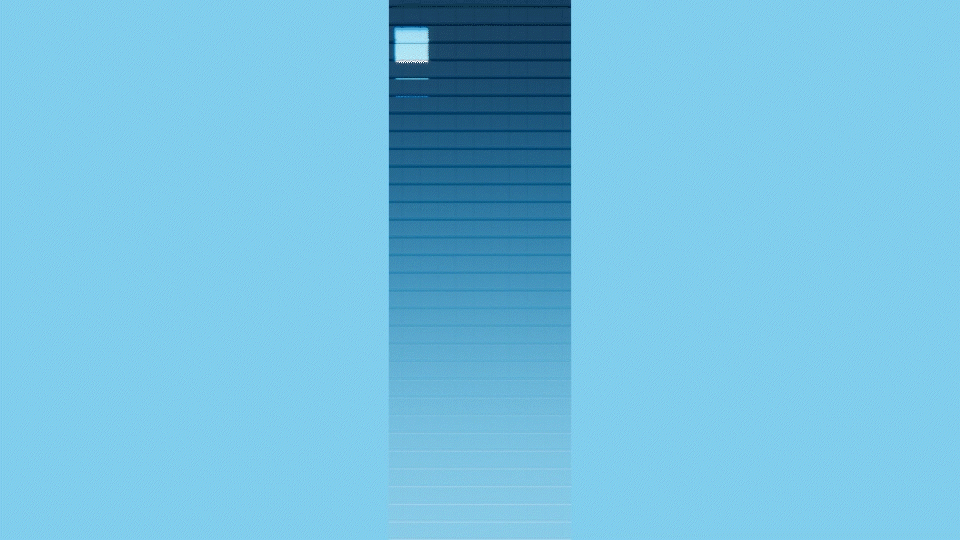